

(2) We design a quality and robust image preprocessing algorithm that prepare and preprocess the unfiltered images for the CNN model and this greatly has a very strong impact on the performance accuracy of our age and gender classifiers. The novel CNN approach addresses the age and gender labels as a set of discrete annotations and train the classifiers that predict the human’s age group and gender. The contributions of this work are summarized as follows: (1) We propose a model that uses CNN architecture to predict the age group and gender of human’s faces from unfiltered real-world environments. The pipeline of our framework for age group and gender. Figure 1 displays the overall idea of the proposed model. We formulate the age and gender classifications task as a classification problem in which the CNN model learns to predict the age and gender from a face image. In light of the current works in age and gender classification and encouraging signs of progress in deep learning and CNN, we therefore propose a novel end-to-end deep learning-based classification model that predicts age group and gender of unfiltered in-the-wild facial images. Recently, deep learning-based methods have shown encouraging performance in this field especially on the age and gender classification of unfiltered face images. The images in these categories have some variations in appearance, noise, pose, and lighting which may affect the ability of those manually designed computer vision methods to accurately classify the age and gender of the images. These conventional hand-engineered methods relied on the differences in dimensions of facial features and face descriptors which do not have the ability to handle the varying degrees of variation observed in these challenging unconstrained imaging conditions.

Many of those methods are handcrafted which perform unsatisfactorily on the age and gender predictions of unconstrained in-the-wild images. Over the past years, a lot of methods have been proposed to solve the classifications problem. Age and gender predictions of unfiltered real-life faces are yet to meet the requirements of commercial and real-world applications in spite of the progress computer vision community keeps making with the continuous improvement of the new techniques that improve the state of the art. However, several issues in age and gender classification are still open problems. Among these features, age and gender classification can be especially helpful in several real-world applications including security and video surveillance, electronic customer relationship management, biometrics, electronic vending machines, human-computer interaction, entertainment, cosmetology, and forensic art. Human’s face contains features that determine identity, age, gender, emotions, and the ethnicity of people.
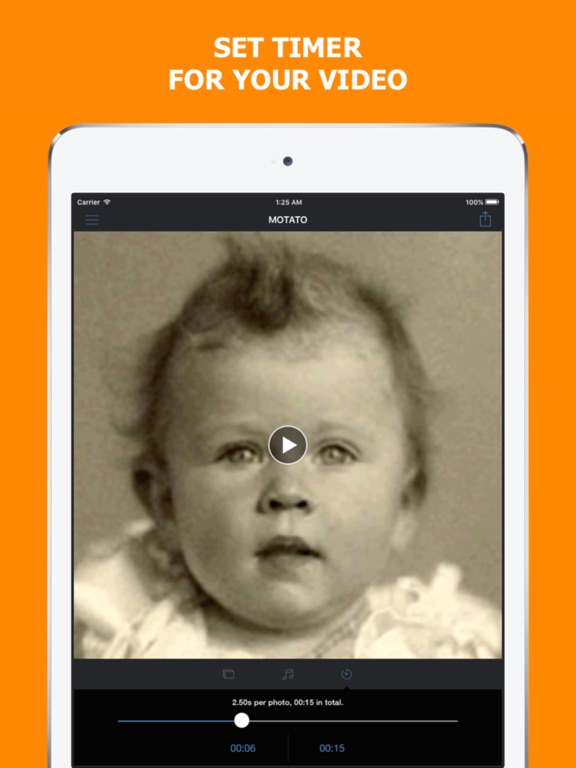
Introductionįacial analysis has gained much recognition in the computer vision community in the recent past. It improves over the best-reported results by 16.6% (exact accuracy) and 3.2% (one-off accuracy) for age group classification and also there is an improvement of 3.0% (exact accuracy) for gender classification. The experimental results, when analyzed for classification accuracy on the same OIU-Adience benchmark, show that our model obtains the state-of-the-art performance in both age group and gender classification. Technically, our network is pretrained on an IMDb-WIKI with noisy labels and then fine-tuned on MORPH-II and finally on the training set of the OIU-Adience (original) dataset. Particularly, we address the large variations in the unfiltered real-world faces with a robust image preprocessing algorithm that prepares and processes those faces before being fed into the CNN model. The feature extraction extracts feature corresponding to age and gender, while the classification classifies the face images to the correct age group and gender. The two-level CNN architecture includes feature extraction and classification itself.

In this work, we propose a novel end-to-end CNN approach, to achieve robust age group and gender classification of unfiltered real-world faces. More recently, Convolutional Neural Networks (CNNs) based methods have been extensively used for the classification task due to their excellent performance in facial analysis. However, the traditional methods on the unfiltered benchmarks show their incompetency to handle large degrees of variations in those unconstrained images. Significant improvements have been made in this research area due to its usefulness in intelligent real-world applications. Age and gender predictions of unfiltered faces classify unconstrained real-world facial images into predefined age and gender.
